1. Introduction
Several people die by accidents, including drowning in Iran every year. Drowning is a preventable cause of mortality. It is referred to as the death within 24 h after suffocation in the water, whereas near-drowning denotes survival beyond 24 h after the event regardless of the outcome [1]. Drowning is often an overlooked public health problem. According to the latest report by the World Health Organization, the estimated global mortality rate of drowning is about 400000 people annually [2]. This fact makes drowning the third leading cause of death due to unintentional injury after car crashes and falling, 97% of which occur in low- and middle-income countries, which is a serious problem for these countries [3].
Most of the deaths in Iran are due to road traffic accidents, burns, and falls, but drowning in the northern areas of Iran is the second leading cause of death caused by unintentional injuries [4]. More than half of drowning deaths occur in children younger than 15 years [4]. Studying the history of drowning in countries with high-income levels reveals that the devastating impact of drowning on public health can be substantially mitigated and prevented [5]. Although drowning is one of the significant threats to public health, it is preventable by several appropriate measures [6]. Effective prevention methods of drowning include identifying the factors involved in drowning, followed by policymaking and planning based on these risk factors [7].
Drowning occurs during daily or recreational activities, such as swimming, boating, fishing, etc. People living in different areas may also be exposed to various causes of drowning, such as sea, lake, river, canal, pond, pool, bathtub, and so on [8]. Predisposing factors, like high-risk behaviors (alcohol consumption and no use of lifesaving equipment), are also different in various parts of the world, possibly influenced by cultural issues [9]. Policymaking, formulation, and implementation of effective programs to prevent this serious problem require more studies [7]. However, there have been few studies on drowning in Iran. Planners and community managers need some indicators, by which they can measure the burden of injury in the society to promote health and also the proper distribution of health facilities among the people [10].
Estimating the future trend is an essential factor for managers, especially those in the health sector, to make decisions [11]. In this regard, this study attempted to predict the drowning rate in Iran using Autoregressive Integrated Moving Average (ARIMA) forecasting model. There were two main questions in this study:
1. How is the trend of death rates caused by drowning in Iran in the coming years?
2. To what extent the ARIMA model can predict this trend appropriately?
2. Materials and Methods
This research was a longitudinal study using the time series data of drowning deaths during 2005-2017obtained from the Iranian Legal Medicine Organization. Given that the time series forecasting can lose its prominence in the long run and sometimes is affected by new and frequent variables [12], the forecast horizon of this study was a medium-term horizon until 2022. The main application of the time series analysis is forecasting. There are several methods for time-series forecasting and the ARIMA method is one of the best models [13], which is based on the Box-Jenkins model [14]. In this model, univariate time series forecasting is performed by statistical modeling. It consists of four stages of model identification, estimation, recognition, and forecasting [15]. The Box-Jenkins models include Autoregressive (AR) model, Moving Average (MA) model, Autoregressive Moving Average (ARMA) model, and ARIMA model that are explained here.
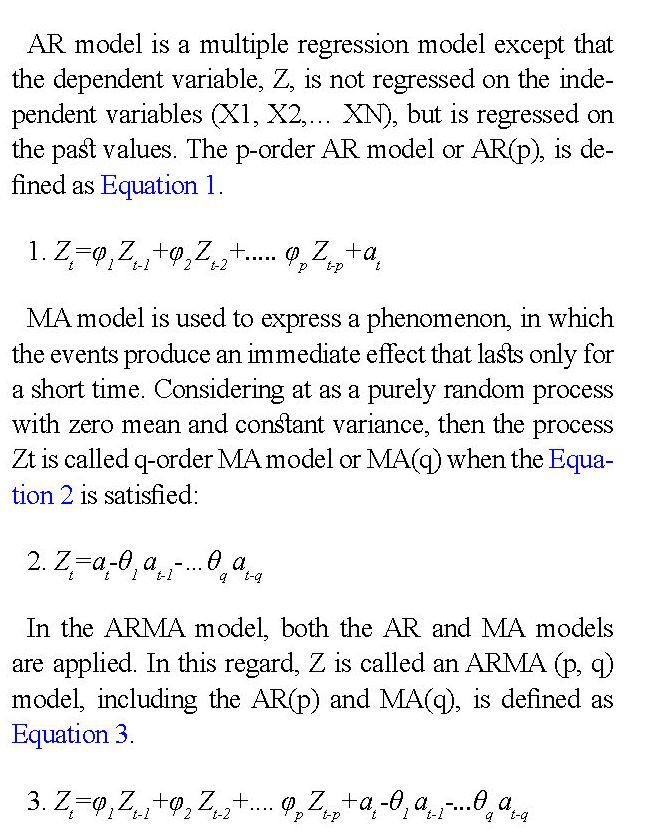
Now we discuss the ARIMA model. Previous models are based on the stationary time series, such that the mean and variance of time series are constant and their covariance remains unchanged over time. However, many time series are non-stationary so they are stacked. If a time series becomes stationary after d times differencing and then we model it with the ARMA (p, q) model, the prime time series is the ARIMA (p, d, q) time series, where p is the number of autoregressive terms, q is the number of moving average terms, and d is the number of differencing transformations for time series to get stationary [16].
3. Results
Figure 1 shows the time series of drowning deaths frequency per the month of incidence, between April 2005 and April 2017. During this period, the lowest number of drowning deaths happened in March 2017, with 19 deaths and the highest number in June 2005, with 251 deaths. A total of 14127 people in Iran died during this period due to drowning, with an average death toll of 1086 people per year. The time series showed that from 2005 to 2009, the trend of drowning was decreasing. From 2009 to 2012, this trend was increasing, and from 2012 to 2017, it was relatively constant with decreasing tendency. Significant differences were found for drowning deaths in different seasons, with the most reported rate in summer (July and August).
Table 1 presents the number of drowning deaths by province and gender. In the last year of the study period (2017), the highest number of deaths was recorded in Khuzestan Province, with 161 deaths, and the lowest number was related to the South Khorasan Province with one death. Moreover, the number of drowned men was 858, whereas the number of drowned women was 168, which indicates that men had a higher rate of drowning death than women. The Mean±SD ages of the drowned men and women were 36±4.25 and 39±5.36 years, respectively.
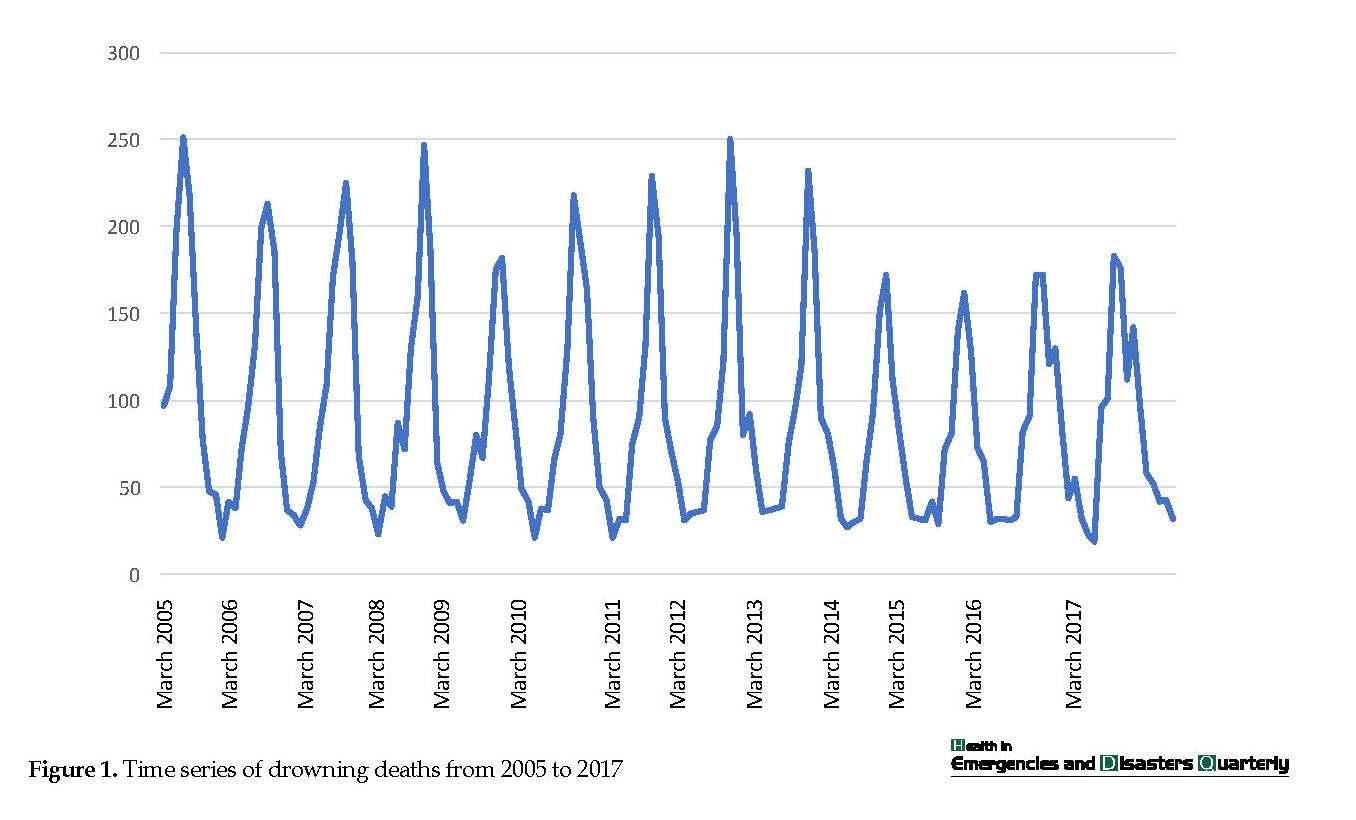
The time series of drowning deaths becomes stationary, after one differencing. Figures 2 and Figures 3 show the Autocorrelation Function (ACF) and Partial Autocorrelation Function (PACF) plots of the drowned people. In the next step, the proper model was identified for the data. The analysis of ACF and PACF showed that the most appropriate model for the drowning deaths was the ARIMA model (4.1.3), ie, a differencing transformation was needed by the time series (d=1) and then it was modeled by an ARMA (4.3) model. The least-squares estimation method was used to estimate the model coefficients. The values of the standard error model were used for determining the best coefficient of the Mean Absolute Percentage Error (MAPE) index, which is shown in Equation 4:

After choosing a specific ARIMA model and estimating its parameters, we examined its goodness of fit, because another ARIMA model may have a better fit for the data. One simple test to check its goodness of fit is that the residuals from this model must look like white noise, with a normal distribution, constant variance, and zero mean. Figure 4 shows the histogram of the residuals. The results showed that the residuals were white noise for the number of drowning events. One important criterion for determining the correct fit of the model is the absolute MAPE index. This index has been used in various studies and shows the percentage of deviation of the predicted values from the actual values [17, 18].
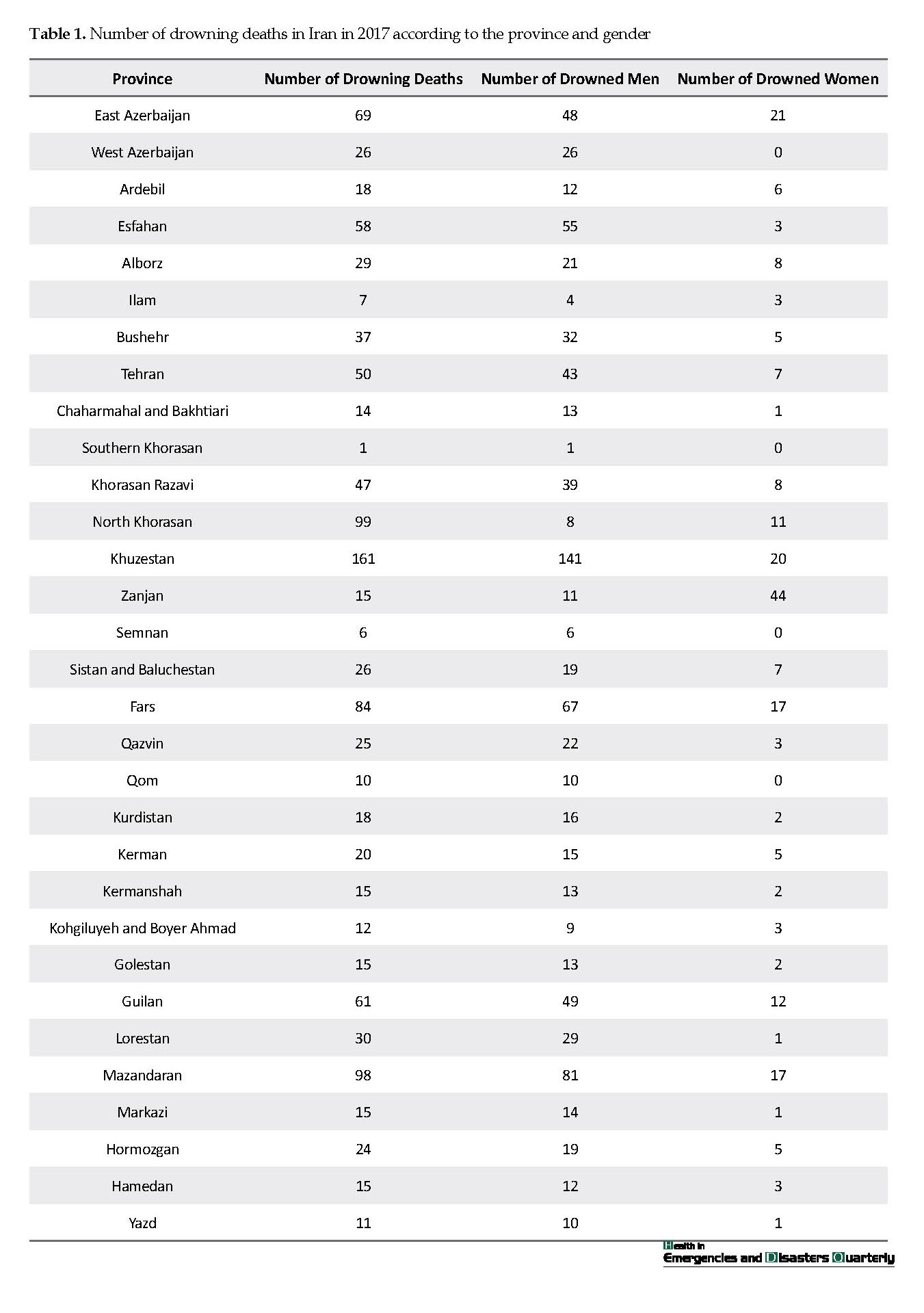
The predicted values using time series models for the next 5 years are presented in Table 2. The predicted annual values are the sum of the predicted values for the years 2018 to 2022. That is, the predicted values for the year 2018 are obtained from the predicted values for the 12 months of this year. Values indicated the beginning of a decreasing trend in the number of drowning deaths in Iran.
4. Discussion
Several people die by accidents and natural disasters every year in Iran. The Caspian Sea, Persian Gulf, and Oman Sea, as well as water resources and water recreations available in Iran, have increased the risk of drowning for Iranians. It has shown that the trend of drowning deaths was decreasing from 2012 to 2018, which indicates the beginning of an upward movement to reduce the risks of drowning. Accidents and disasters, in addition to the adverse effects on the injured person, exert economic and social burden on society.
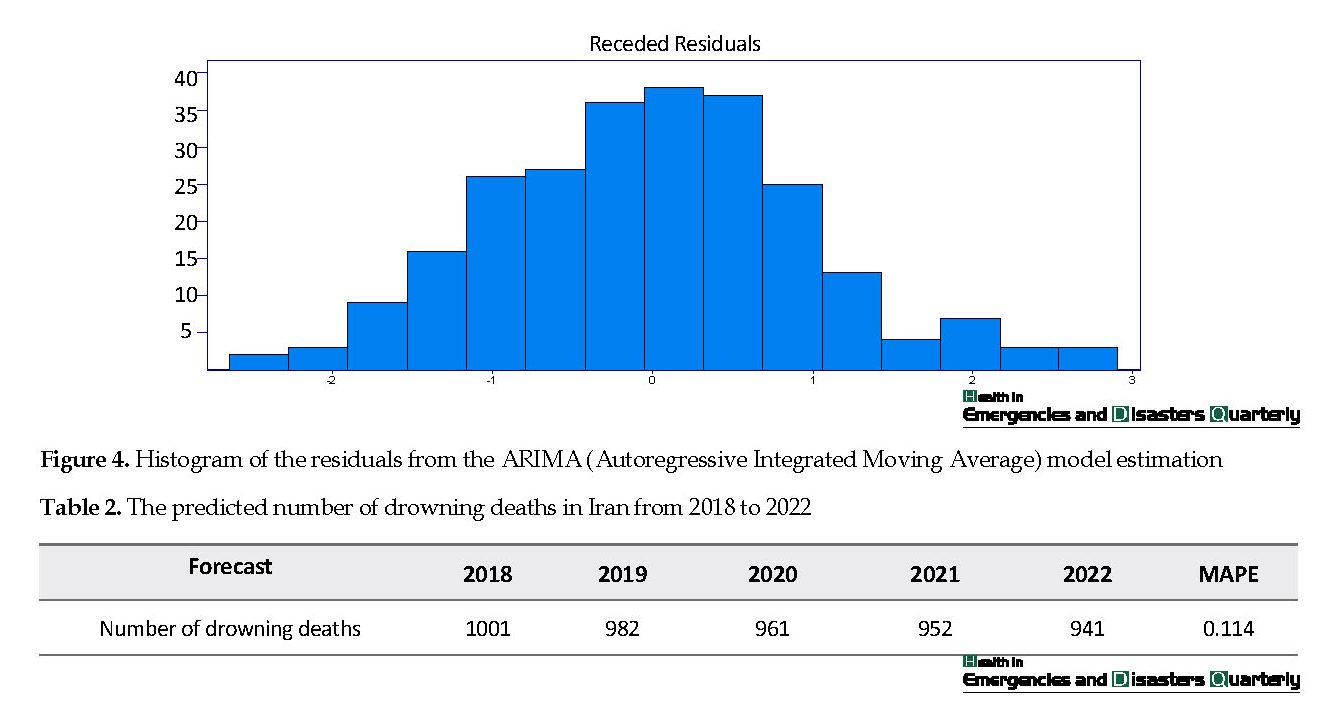
The downward trend of drowning deaths in Iran can be associated with the controlled deaths caused by drowning in this country. Davoodi et al. (2008) study on the trend of drowning deaths in Iran shows that this trend has declined over the years [19]. The leading cause of the reduced drowning mortality rate can be due to reduced exposure, having preventive programs, or improvement of the recreational areas for swimming.
The results showed that the number of drowned men was much more than women. This result may be because men have more access to places for swimming, and they have risk-taking and high-risk behaviors, such as swimming alone. Iranian women, on the other hand, are socially and culturally limited for swimming in public and open places, so no cases of drowned women were observed in West Azerbaijan, South Khorasan, Semnan, and Qom provinces in 2017.
In the present study, there was a significant difference in the number of drowned people in terms of seasons. Most cases of drowning occurred in summer (June and August). People are attracted to swimming, water sports and using available natural waters in summer due to the excessive heat, which results in the increased number of drowning cases. Cohen et al. in their study also indicated the impact of seasonal changes and climate factors on drowning [2].
The results of our study revealed that statistical methods with high accuracy could be used to predict accidents and disasters. Hosseini Kebria et al. [20] used statistical methods to predict the occupational accidents of oil Refinery sector. Their study showed that the fuzzy logic model could accurately predict occupational accidents in the workplace. Omidi and Omidi [21] showed that statistical methods have high accuracy for the prediction of accident-related traumatic injury rates, and these methods can be used as highly guaranteed methods for estimating future accidents.
5. Conclusion
Our results demonstrated that the number of drowned people in Iran is decreasing, and in the future, this trend will be decreased with a steeper slope. Considering this decreasing trend, we can investigate the weaknesses and strengths of the provinces of Iran in reducing the number of drowning deaths by surveying the drowning-related death rate in different provinces and identifying the most successful provinces in reducing the rate of drowning death. It is suggested that in further studies, by categorizing provinces, the drowning mortality trend for each province be examined to determine its decreasing and increasing trends separately.
Ethical Considerations
Compliance with ethical guidelines
All ethical principles were considered in this article.
Funding
This research did not receive any specific grant from funding agencies in the public, commercial, or not-forpro it sectors.
Authors' contributions
Writing text and doing calculations: Mohammad Reza Omidi; Interviewing and distributing questionnaires: Meisam Jafari Eskandari and Sedigh Raissi; Data collection and analysis: Amir Abbas Shojaei.
Conflict of interest
The authors declared no conflict of interest.
References
Macintosh I, Austin S. Management of drowning in children. Paediatrics and Child Health. 2017; 27(9):415-9. [DOI:10.1016/j.paed.2017.05.008]
Cohen RH, Matter KC, Sinclair SA, Smith GA, Xiang H. Unintentional pediatric submersion-injury-related hospitalizations in the United States, 2003. Injury Prevention: Journal of the International Society for Child and Adolescent Injury Prevention. 2008; 14(2):131-5. [DOI:10.1136/ip.2007.016998] [PMID]
Lee DH, Park JH, Choi SP, Oh JH, Wee JH. Clinical characteristics of elderly drowning patients. The American Journal of Emergency Medicine. 2019; 37(6):1091-5.
Divesalar A. A survey on the drownings of Mazandaran province in 2007. Iranian Journal of Forensic Medicine. 2010; 16(1):7-13.
Tanaka N, Kinoshita H, Jamal M, Takakura A, Kumihashi M, Miyatake N, et al. Detection of chlorine and bromine in free liquid from the sphenoid sinus as an indicator of seawater drowning. Legal Medicine. 2015; 17(5):299-303. [DOI:10.1016/j.legalmed.2015.08.005] [PMID]
Nnafie A, de Swart HE, Calvete D, Garnier R. Effects of sea level rise on the formation and drowning of shoreface-connected sand ridges, a model study. Continental Shelf Research. 2014; 80:32-48. [DOI:10.1016/j.csr.2014.02.017]
Usumoto Y, Sameshima N, Hikiji W, Tsuji A, Kudo K, Inoue H, et al. Electrolyte analysis of pleural effusion as an indicator of drowning in seawater and freshwater. Journal of Forensic and Legal Medicine. 2009; 16(6):321-4. [DOI:10.1016/j.jflm.2008.12.028] [PMID]
Kakizaki E, Sonoda A, Sakai M, Yukawa N. Simple detection of bacterioplankton using a loop-mediated isothermal amplification (LAMP) assay: First practical approach to 72 cases of suspected drowning. Forensic Science International. 2018; 289:289-303. [DOI:10.1016/j.forsciint.2018.05.035] [PMID]
Pierucci G, Merlano F, Chen Y, Sturini M, Maraschi F, Profumo A. Haematic silicon in drowning. Journal of Forensic and Legal Medicine. 2016; 39:22-6. [DOI:10.1016/j.jflm.2016.01.007] [PMID]
Bäckman A, Hollenberg J, Svensson L, Ringh M, Nordberg P, Djärv T, et al. Drones for Provision of Flotation Support in Simulated Drowning. Air Medical Journal. 2018; 37(3):170-3. [DOI:10.1016/j.amj.2018.01.007] [PMID]
Seematter-Bagnoud L, Fustinoni S, Dung DH, Santos-Eggimann B, Koehn V, Bize R, et al. Comparison of different methods to forecast hospital bed needs. European Geriatric Medicine. 2015; 6(3):262-6. [DOI:10.1016/j.eurger.2014.09.004]
Matyjaszek M, Riesgo Fernández P, Krzemień A, Wodarski K, Fidalgo Valverde G. Forecasting coking coal prices by means of ARIMA models and neural networks, considering the transgenic time series theory. Resources Policy. 2019; 61:283-92. [DOI:10.1016/j.resourpol.2019.02.017]
He Z, Tao H. Epidemiology and ARIMA model of positive-rate of influenza viruses among children in Wuhan, China: A nine-year retrospective study. International Journal of Infectious Diseases. 2018; 74:61-70. [DOI:10.1016/j.ijid.2018.07.003] [PMID]
Zhang L, Lin J, Qiu R, Hu X, Zhang H, Chen Q, et al. Trend analysis and forecast of PM2.5 in Fuzhou, China using the ARIMA model. Ecological Indicators. 2018; 95:702-10. [DOI:10.1016/j.ecolind.2018.08.032]
Qin M, Li Z, Du Z. Red tide time series forecasting by combining ARIMA and deep belief network. Knowledge-Based Systems. 2017; 125:39-52. [DOI:10.1016/j.knosys.2017.03.027]
Barak S, Sadegh SS. Forecasting energy consumption using ensemble ARIMA-ANFIS hybrid algorithm. International Journal of Electrical Power & Energy Systems. 2016; 82:92-104. [DOI:10.1016/j.ijepes.2016.03.012]
Rabiee I, Mahdavi I, Bagherpour M, Tavakkoli-Moghaddam R. Applying Forecasting Models Through Estimate at Completion Cost of Project in Using Earned Value Analysis. Journal of Industrial Engineering. 2011; 45(2):145-57.
Gorgini M, Golestani S, Hajabbasi F. A comparison of the predictive ability of VAR, ARIMA and Artificial Neural Network (ANN) Models: OPEC’s oil demand. Iranian Energy Economics. 2012; 1(4):145-68.
Kiakalayeh AD, Mohammadi R, Ekman DS, Chabok SY, Janson B. Unintentional drowning in northern Iran: A population-based study. Accident, Analysis and Prevention. 2008; 40(6):1977-81. [DOI:10.1016/j.aap.2008.08.008] [PMID]
Hosseini Kebria SS, Mohammadi Golafshani E, Kashefi alasl M, jozi SA. Predicting the occupational accidents of Tehran’s oil Refinery based on HSE using fuzzy logic model. Iran Occupational Health. 2014; 11(6):43-54.
Omidi N, Omidi MR. Estimating accident-related traumatic injury rate by future studies models in Semnan Province, Iran. Health in Emergencies and Disasters. 2018; 3(4):191-8. [DOI:10.32598/hdq.3.4.191]